How Expensify’s open-source program is powering OpenAI’s next-gen AI engineering benchmarks
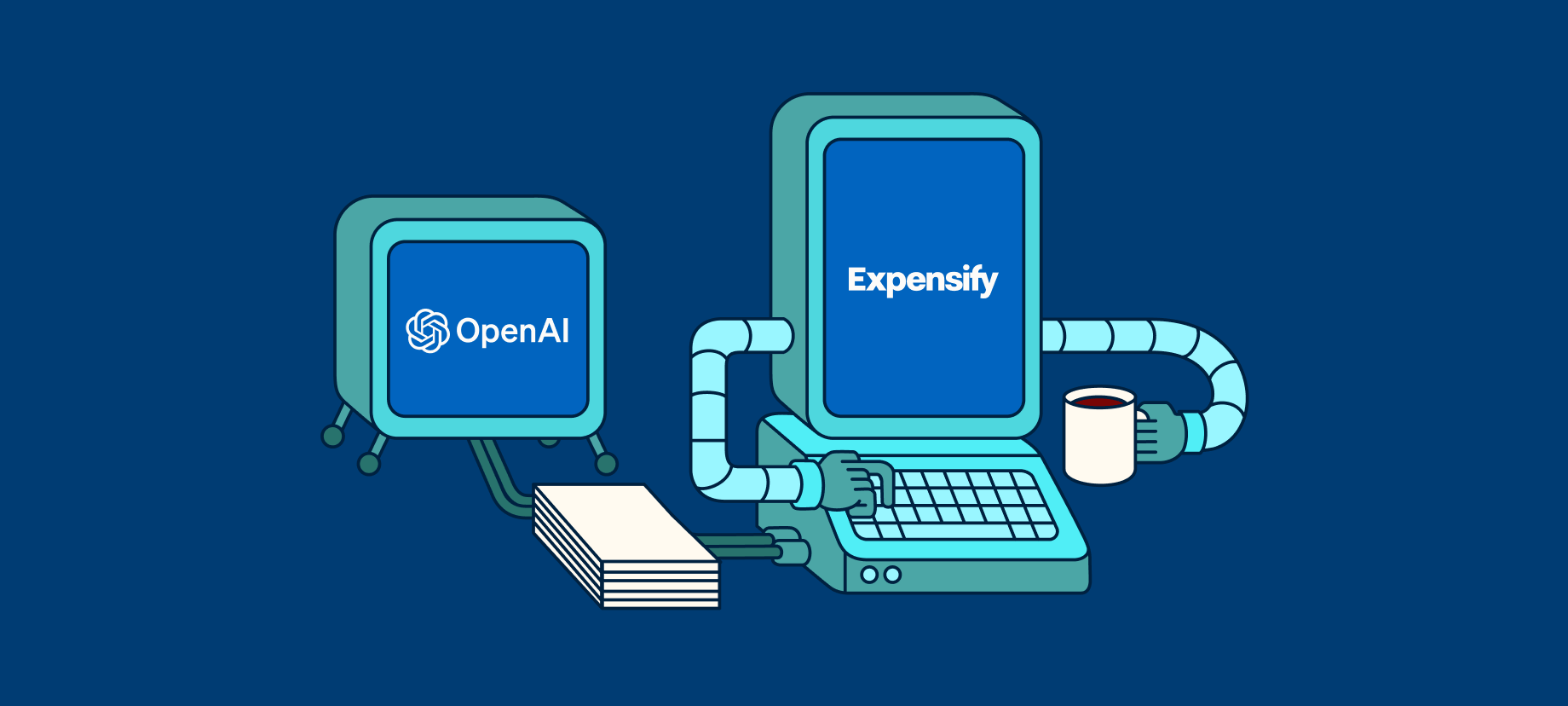
The rapid rise of large language models (LLMs) has opened up exciting new possibilities for AI-driven software development. But how well can these models tackle real-world engineering tasks?
That question led to the creation of SWE-Lancer—a benchmark developed by OpenAI that evaluates LLMs using real freelance software tasks. Let's explore how Expensify’s open-source repository and freelance program enabled the creation of SWE-Lancer.
SWE-Lancer: A real-world testbed for AI in software engineering
Most AI coding benchmarks focus on isolated code snippets or theoretical problems. SWE-Lancer is different—it evaluates LLMs on 1,488 real-world software tasks, all sourced from Expensify’s freelance jobs and the open-source repository.
These tasks range from simple bug fixes ($250 payouts) to complex feature implementations ($32,000 payouts), covering:
Frontend & backend development
API integrations
Full-stack debugging
Software architecture decision-making
By leveraging Expensify’s open-source development process—which encapsulates the intricate challenges of real-world engineering workflows from initial triage to testing and deployment—SWE-Lancer provides the most realistic AI software engineering benchmark to date.
Mapping AI performance to real economic value
One of SWE-Lancer’s most significant innovations is mapping AI coding performance to real-world money. Since each task in the benchmark has an actual payout from Expensify, researchers can measure AI success not just in terms of accuracy but in dollars earned.
For example, the best-performing LLM in the study, Claude 3.5 Sonnet, earned $403,000 out of a possible $1,000,000.
This unique approach allows researchers to estimate AI’s ability to solve real tasks l on the freelance job market—helping to predict how automation may shape software engineering careers in the future.
A more rigorous evaluation standard
Existing AI coding benchmarks often rely on unit tests, which can be easily gamed by models that memorize patterns instead of understanding the problem. SWE-Lancer takes a different approach by emphasizing:
End-to-end tests: Simulating real user workflows to ensure AI solutions work in production
Real-world challenges: Leveraging authentic issues from the Expensify app ensures that models tackle genuine, production-level problems with real business impact
Full-stack evaluation: AI models must reason across multiple layers of an application, rather than solving isolated coding problems
By raising the bar for AI evaluation, SWE-Lancer provides deeper insights into where AI can truly contribute to software development.
AI as a software manager
Beyond coding, SWE-Lancer also evaluates whether LLMs can function as software managers. Within Expensify’s freelance process, one step requires a hiring decision to be made after reviewing submitted proposals. This provided the basis for SWE Manager tasks, where models:
Review multiple software proposals
Select the best implementation strategy
Are graded based on how well they match real human engineering manager decisions
Surprisingly, LLMs performed better at management tasks than direct coding, suggesting that AI may first integrate into software teams as an advisor rather than a coder.
AI and the future of freelance work
Expensify is one of the few companies actively integrating freelance engineering into an open-source development model. This model allows an early glimpse into how AI could impact the freelance economy:
Will AI replace freelance engineers, or augment them?
Can LLMs bridge skill gaps for underrepresented developers?
Might engineering managers lean on LLMs as advisors?
With an open-source development process, Expensify is helping the AI community explore these questions.
A bold experiment with real-world implications
Expensify’s contributions to SWE-Lancer represent a real-world experiment in AI-driven software engineering. With our open-source app and freelance program, Expensify has helped create the most realistic AI coding benchmark to date.
This research is just the beginning. As AI models continue to evolve, Expensify’s open-source development model could serve as a blueprint for the future of AI-assisted engineering teams.
FAQs
-
SWE-Lancer is an AI programming benchmark developed by OpenAI that helps to measure the ability of LLMs to complete software tasks including code generation, refactoring, and documenting code.
-
Our freelance program allows individual contributors to complete paid tasks while contributing to Expensify’s open source app. We are welcoming freelancers from all over the world to take part in shaping the future of financial collaboration.
-
Expensify's open-source program provides OpenAI's SWE-Lancer benchmark with 1,488 real-world software engineering tasks, ranging from bug fixes to complex feature implementations. These tasks, which come with actual monetary payouts ($250-$32,000), allow researchers to evaluate AI models' performance in terms of real economic value and practical engineering capabilities.
What makes this contribution particularly valuable is that it offers production-level problems, and management task evaluation—providing a much more realistic benchmark than traditional coding tests that rely on isolated snippets or theoretical problems.